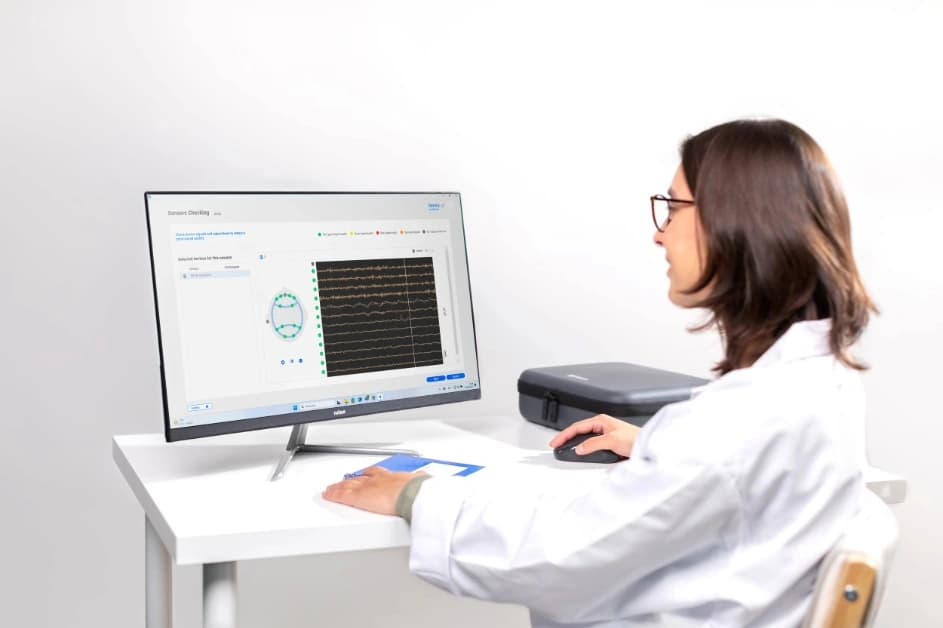
EEG Datasets - New
EEG Sleep Dataset
This project aims to bridge the gap between sleep monitoring (PSG) and wearable EEG technology.
The dataset includes 128 nights of simultaneous recordings from a Micromed PSG system and a Bitbrain wearable EEG headband Ikon Sleep.
Sleep stages were labelled through the consensus of three independent experts. We utilized a deep learning model to automatically label the sleep stages, obtaining a match > 85% between the human-consensus labels and the network labels.
The dataset contains:
- Raw and labelled PSG and wearable EEG data.
- Human-consensus and AI-generated sleep stage labels for both systems.
EEG Textile Dataset
This paper introduces the first garment capable of measuring brain activity with accuracy comparable to state-of-the-art dry EEG systems.
The first open-access dataset uses textile-based EEG (Bitbrain Ikon EEG headband), connected to a mobile EEG amplifier and tested against a standard dry-EEG system.
The innovation lies in an EEG sensor layer made entirely of threads and smart textiles, without metal or plastic. The textile-based system offers better comfort while results show comparable recordings, though more artifacts under poor conditions.
Gel, Water and Dry EEG Systems Dataset
This study examined whether EEG correlates of natural reach-and-grasp actions could be decoded using mobile EEG systems.
Reaching and grasping are vital for interaction and independence. While EEG studies have identified neural correlations, their applicability to mobile EEG systems for home use remains uncertain.
The dataset includes data from Bitbrain mobile EEG systems, the water-based EEG Versatile cap and the dry-sensor EEG Hero headset. Gel-based recordings from a laboratory environment served as a gold standard.
Results confirmed that EEG correlates were identifiable using mobile systems. A multiclass decoding approach revealed decodable low-frequency time domain (LFTD) correlates, with accuracies of 62.3% for the water-based cap, 56.4% for the dry-sensor headset, and 61.3% for the gel-based system.